Jobs in Artificial Intelligence Salary
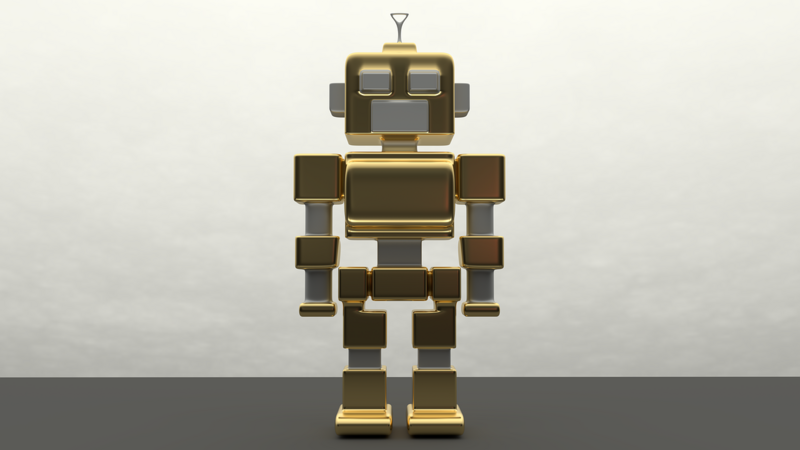
Artificial Intelligence jobs are on the increase as the salary is appealing.
Inside Nvidia’s 13,000 square foot AI robotics research lab in Seattle, a small team of researchers is hard at work building the company’s artificial intelligence-powered future. Next to a kitchen worktop, a robotic arm lifts a tin of Spam and puts it in a drawer. The arm has also learned how to clean the dining table and, if you ask nicely, it can help you cook a meal. This, right here, is the first tentative step in Nvidia’s ambitious artificial intelligence master plan.
Opened at the start of the year, the lab currently employs 28 people, with capacity for 50 research scientists, faculty advisors, and interns when operating at full-tilt. Led by renowned roboticist Dieter Fox, senior director of robotics research at Nvidia and a professor at the University of Washington, the lab is aiming to develop the next generation of robots that can safely work alongside humans, possibly transforming industries like manufacturing, logistics, and healthcare in the process. It’s one of the many grand challenges for artificial intelligence – a sci-fi vision of robots that aren’t just good at very specific tasks, but capable of behaving as if almost human.
But the robot is also a somewhat elaborate muscle flexing exercise. It’s an eye-catching demonstration of what Nvidia’s hardware and software can do today and could do in the future – and a bid by the company to stay one step ahead of an increasingly speedy competition.
Optimising graphics processing unit, or GPUs, for AI back in 2005 must go down as one of the smartest business decisions in technology hardware history. But now it’s on top, and faced with increasing competition, consolidating that position will be one of the toughest tests in Nvidia’s 25-year history.
Nvidia’s latest lab has been kitted out with equipment and real life environments that robots are expected to encounter in the real world. The first of these scenarios is a humble kitchen. The robot assistant integrates AI and deep learning techniques to detect and track objects, understand the relative positions of doors and drawers, and open and close them to access specific items. “In the past, robotics research has focused on small, independent projects rather than fully integrated systems,” Fox says. “We’re bringing together a collaborative, interdisciplinary team of experts in robot control and perception, computer vision, human-robot interaction, and deep learning.”
The team in Seattle is being assisted by around 60 researchers spread across Nvidia’s other research hubs in Santa Clara, Eastern Massachusetts, Toronto and Tel Aviv. The multidisciplinary approach has a fiendishly difficult goal: Nvidia is trying to show that its artificial intelligence hardware and software can transform robots from what they are today – very accurate positioning machines that dumbly follow regimented instructions – into dynamic and flexible machines that can safely work alongside humans.
Samim Wagner, an AI researcher for Google in Berlin, believes one of the main reasons Nvidia is carrying out its own research is to help it build better AI hardware that it can then sell on to others. “In order to build feasible and competitive hardware for machine learning, Nvidia is strategically forced to do high quality machine learning research,” he says. “The company’s traditional connections with the games and entertainment industries provide it with a focus-point for its machine learning research, which is key for success.” Which helps explain the robot – and a host of other oddball experiments taking place in Nvidia’s research laboratories.
“Now that we have deep learning it’s possible to make robots interact with their environment,” says Bill Dally, Nvidia’s chief scientist and senior vice president of research, in reference to the robot kitchen helper. “Now that we can build perceptual systems, we can build robots that don’t count on the car being in the exact same spot each time.”
As with Nvidia’s experiments with robotics, deep learning has enabled AI systems to tackle problems that were previously impossible. Through its trial-and-error technique, breakthroughs have been made in virtual assistants, computer vision, language translation, chatbots and facial recognition. And, as with so much in artificial intelligence, such breakthroughs rely heavily on clever little GPUs.
Nvidia’s AI journey started at Joanie’s Cafe in Palo Alto, California, in 2010. “We got into AI after a breakfast meeting I had with Andrew Ng,” says Dally. At that breakfast, Ng, a well known AI researcher who was working with Google Brain at the time, explained how Google was training AI systems to recognise photos of cats with the help of 16,000 central processing units, or CPUs. After pointing out next to no one has 16,000 CPUs at their disposal, Dally laid down a challenge. “I bet we could do this with way fewer GPUs,” he said to Ng.
Shortly after the encounter, Dally asked Nvidia researcher Bryan Catanzaro to team up with Ng and do just that. “We achieved roughly the same performance with 48 GPUs that they had done with 16,000 CPUs,” says Dally. “At the time it was really clear to me that this was going to transform everything.”
From a bet about recognising cats, Nvidia’s AI business has exploded. The company doesn’t disclose exactly how much it is currently investing into the field, but filings reveal it spent a total of $1.8 billion (£1.4b) on all research in the last financial year. Key areas of investment were gaming, AI and automotive. The effectiveness of its GPUs for artificial intelligence projects has created a scramble amongst Nvidia’s competitors, with Intel, Google and even Facebook investing huge sums of money to try and catch up.
In July 2018, Google announced it was developing its own AI chips for on-device machine learning. Google’s bet is on a new kind of chip: a tensor processing unit designed specifically for neural network machine learning. And a separate, equally important tussle is also unfolding. As well as hardware, Nvidia’s code library is also going up against rival frameworks such as Google Tensorflow or Facebook PyTorch. Think of it as deep learning as a service. And, against all the odds, Nvidia is still holding its own.
The reason? As the cat challenge showed, GPUs are brilliant at handling AI tasks. That’s because GPUs are better than CPUs at running the parallel computing problems associated with AI, where many calculations are carried out simultaneously. Parallel computing is particularly prevalent in neural networks because they are designed to work in a similar way to animal brains, which can do many tasks at the same time, or in parallel. Eventually, Nvidia wants to be the one building the artificial brains inside every phone, computer, robot and autonomous car.
Led by CEO Jen-Hsun Huang, the company effectively preempted the AI boom back in 2005 when it created software that allowed its GPUs to process the millions of minuscule computations that would eventually be required by modern AI. Its GPUs are now in data centres around the world, powering AI tasks for thousands of businesses. Between 2014 and 2018, the company was boosted by a 524 per cent increase in revenue for its data centre-optimised chips.
“We’re the supplier to the whole world,” says Dally. “Everyone’s training their deep neural networks on Nvidia GPUs,” he continues, adding that the company’s T4 chip is widely used for inference tasks. Facebook and Google use Nvidia’s platform to power the AI features on their platforms. Almost all the driverless car companies are using Nvidia technology. The company has also signed deals with Tencent, Alibaba, and Baidu.
As with the robot in the kitchen, Nvidia’s other AI experiments appear similarly eclectic and oddball. It’s built a creative neural network, known as a generative adversarial network, or GAN, that’s scarily good at creating hyper-realistic images of human faces. In a paper released in December 2018, the company showed just how clear its GAN faces are compared to GAN faces from four years ago.
Faces aren’t the only thing that Nvidia’s GANs can create, or fake. Nvidia has also developed a translation network that can turn photos taken in winter into beautiful summery scenes. Got a rainy photo of your wedding you want brightening up? Nvidia’s AI can make it look just like the sun was shining all along. And it doesn’t stop there. Nvidia showed off the world’s first video game demo to use AI-generated graphics at the end of last year. The demo was a driving simulator that was built by combining AI-generated visuals with a standard video game engine.
But there have been bumps in the road. And they hint at a rougher ride to come. At the start of the year, Nvidia was forced to cut its fourth quarter revenue forecast by $500 million in a move that saw its stock tumble nearly 18 per cent. The cryptocurrency crash that rumbled through much of 2018 was extremely painful for Nvidia’s bottom line, costing it an estimated $23 billion in market value. But it was also a blessing – albeit an expensive one.
For the time being, the adaptability of Nvidia’s GPUs give it an edge over its rivals: they’re integral to data centres, self-driving cars, research labs, cryptocurrency mining rigs – you name it, there’s a GPU in it. But that could soon change. And that’s where Nvidia’s research labs come into play.
“There’s a slew of new AI hardware startups,” says Stephen Merity, an AI research consultant and the founder of a startup in stealth mode. “They may well be a threat but it’s a huge open question. I can’t imagine any of those startups hitting mass scale early on.”
One such startup is UK-based Graphcore, which is now valued at over $1 billion (£760,000) thanks to funding from the likes of BMW, Sequoia, and the founder of Google-owned DeepMind. Graphcore claims its intelligence processing units, which are yet to go into mass production, are 100 times faster than any existing systems. For Nvidia, that could create a financial headache bigger than the cryptocrash.

Latest Jobs
-
- Identity Channel Partner Manager | London
- London
- N/A
-
Identity Channel Partner Manager | London Location: South East UK (commutable to London) We are working with a Cyber Security business who are looking for a Channel Partner Manager to drive and grow relationships across their identity ecosystem. Prior experience working within VARs, distributors, vendors or resellers in the identity space is essential. You must have experience working with technologies such as CyberArk, Sailpoint, Okta etc Responsibilities will include, but not be limited to: Build, maintain and develop strong relationships with channel partners. Work closely with partner sales teams to support growth drive sales opportunities. Identify and onboard new partners while strengthening existing partnerships. Act as the key point of contact for all channel-related activity. If you are an experienced channel professional, with experience in the Identity space and are ready for your next challenge, apply today.
-
- Service Architect- DACH regions
- Germany
- Upto €110,000 plus bonus and benefits
-
Lead Service Architect with the authority and experience to take control of complex, multi-million-euro outsourcing bids. This role is about leading the Service/ solutioning effort, bringing structure to chaos, and driving the entire bid team to deliver winning proposals. The company area a global managed services business working with enterprise and public sector clients, across Cloud, End-User Computing, Digital Workplace, Service Desk, and Network Infrastructure. What You’ll Do: Lead Service/ solution design from qualification to contract. Control bid teams — architects, pricing, delivery, and SMEs. Break down RFPs/RFIs into actionable, costed, client-ready solutions. Present internally and to clients at decision-maker level. Run solution workshops, own the architecture, and shape the financial model. You’ll Need: Experience working as a Service architect, Service Manager or Customer Success Manager R Gravitas to lead and drive teams through high-stakes bids. Deep knowledge of managed services delivery and commercial models. Strong technical grasp: Cloud, Security, EUC, Unified Comms, Service Desk, and more. Experience leading deals across onshore, offshore, and hybrid delivery models.
-
- Deal Architect- DACH region
- Germany
- Upto €110,000 plus bonus and benefits
-
Lead Deal Architect with the authority and experience to take control of complex, multi-million-euro outsourcing bids. This role is about leading the solutioning/ Service effort, bringing structure to chaos, and driving the entire bid team to deliver winning proposals. The company is a global managed services business providing solutions to enterprise and public sector clients, across Cloud, End-User Computing, Digital Workplace, Service Desk, and Network Infrastructure. What You’ll Do: Lead the deal from qualification to contract. Control bid teams — architects, pricing, delivery, and SMEs. Break down RFPs/RFIs into actionable, costed, client-ready solutions. Present internally and to clients at decision-maker level. Run solution workshops, own the architecture, and shape the financial model. Be responsible for the service Wrap and ensuring the Service meets clients requirements You’ll Need: A back ground with IT Services Experience in a similar type of role, for example: Deal, Service, or Solution Architect in ICT outsourcing. Gravitas to lead and drive teams through high-stakes bids. Deep knowledge of managed services delivery and commercial models. Strong technical knowledge: Cloud, Security, EUC, Unified Comms, Service Desk, and more. Experience leading deals across onshore, offshore, and hybrid delivery models.
-
- Pre Sales Lead- IT Services
- Germany
- Upto €100,000 plus benefits
-
As the Pre-Sales Lead (Sales Engineer/ Solution Architect) you will drive large-scale ICT managed services and outsourcing deals (from €0.5M to €20M+). You'll work directly with Business Development and clients to design high-impact solutions across Cloud (Azure, IaaS, SaaS, PaaS), EUC, Unified Comms, Security (SIEM, PAM), Networks, and Smart Workplaces. What You’ll Do: Lead the end-to-end pre-sales cycle — from RFI/RFP to contract. Design innovative, client-specific solutions with technical & commercial impact. Present at CxO level and steer proposal strategies & financial models. Collaborate closely with Portfolio, Service Desk, Field, and Digital Workplace teams. Support deal shaping with strong knowledge of ITIL, SIAM, Automation, and cost analysis. What You’ll Bring: Have strong experience in pre-sales or solution architecture. Experience with €M+ managed service deals. Deep technical expertise in modern ICT stack and enterprise IT services. Strong German (C1) and English communication skills. Certifications: ITIL v3/v4 required; SIAM, ISO20000 desirable.